IHC - CLEO BREAST
End-to-End Pipeline for automatic IHC grading in Breast Cancer
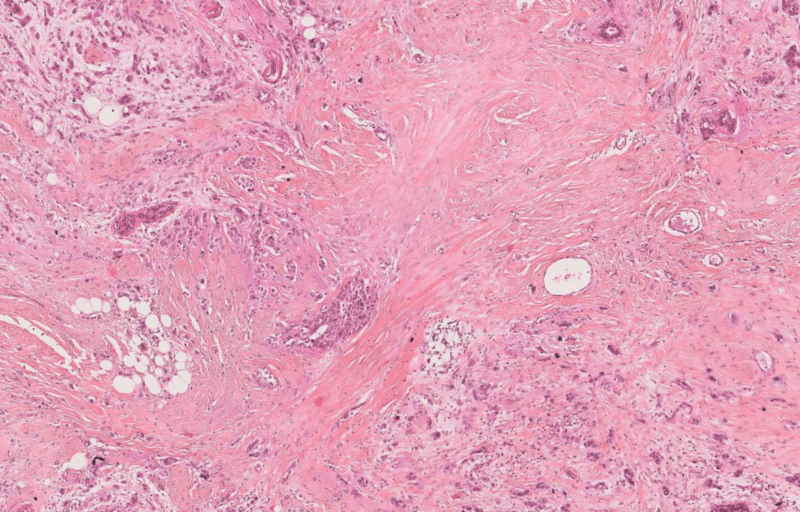
By : Nicolas Nerrienet (Primaa), Clara Simmat (Primaa), Rémy Peyret (Primaa), Samuel Touioui, Marie Sockeel (Primaa), Bastien Jean Jacques (CHU de Caen), Elise Sauvain (CHU de Reims), Stéphane Sockeel (Primaa), Elisabeth Lanteri (Medipath).
Introduction
Immuno-histochemistry (IHC) is a staining process that highlights important biomarkers for assessing prognosis and guiding therapeutic decisions. Grading Ki67 and hormonal receptors in IHC-stained slides involves:
- Identifying invasive carcinoma (IC) regions in hematoxylin-eosin (HE)
- Locating them in associated IHC-stained slides
- Evaluating the presence of target antigens for each nuclei in IC regions by calculating the ratio between the number of positive nuclei and the total number of nuclei.
This process is tedious and time consuming. It has also proven to hold high inter-observer variability.
Artificial intelligence (AI)-based systems could efficiently assist pathologists in a more accurate clinical diagnosis.
- Material & Methods
- Datasets and training
- IC detection. Fine-tuning a HE EfficientNet on IHC.
Fine-tuning a pre-trained EfficientNet trained to predict IC on HE slides on a set of 210 IHCs (KI67 – RE – RP) multi-centric slides.
Control detection. Random Forest classifier to detect controls.
IHC slide often contains « controls » which are used to verify that the staining process succeed on the main tissue. These controls should not be considered for IHC scoring.
Nuclei detection. U-net segmentation on hematoxylin channel.
Inference pipeline
Positive and negative nucleus classification
Experiments & Results
IHC status determination
We tested the end-to-end pipeline presented in the previous section for ~100 slides from ACROBAT dataset. To measure scoring quality, we assessed the correlation coefficient between the ratio assigned by the pathologist and the ratio provided by the algorithm Here are the scatter plot of predictions against ground truth with categories thresholds (with a 5% error margin) for Ki67 and ER.
Discussion
We observe strong correlations (R²=0.78 and 0.86) between the predicted and ground truth IHC slides score. The following table classifies errors for misclassified slides – excluding those falling within a 5% margin of error, indicating their proximity to the true values.
(a) Errors classification. Most errors involve controls being mistaken for tissues of interest, highlighting the necessity for standardization.
Conclusion
In this work, we developed a complete end-to-end pipeline capable of *detecting IC regions directly on IHC stained slides and *scoring IHC using those regions. To the best of our knowledge, this end-to-end tool is the first of its kind as existing solutions need pathologists to select an IC region for the score to be computed in. Our results indicate that the proposed pipeline has a great potential to assist pathologists with their daily routine.