Deep Learning-Driven End-to-End Solution for Automated HER2 Grading in Breast Cancer
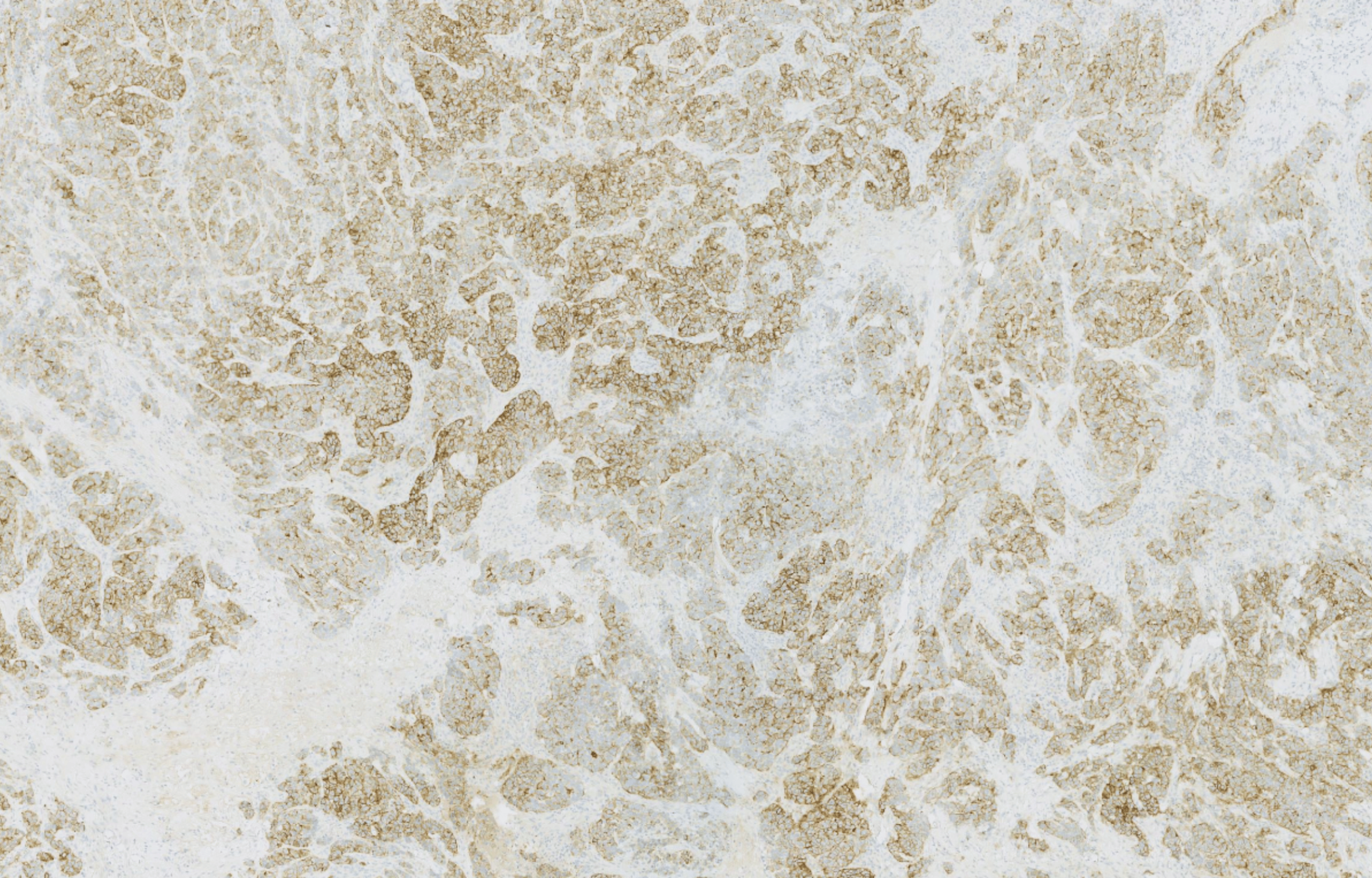
Clara SIMMAT MSc, Rémy PEYRET PhD, Nicolas NERRIENET MSc, Nicolas HAMADOUCHE MD, Elise MARCO-SAUVAIN MD, Bastien JEAN-JACQUES MD, Stéphanie DELAUNAY MD, Elisabeth LANTERI MD, Marie SOCKEEL MD, Stéphane SOCKEEL PhD, Arnaud GAUTHIER MD
Background
HER2 is a key biomarker guiding treatment decisions for breast cancer.
Assessing HER2 expression involves several steps:
- Identifying regions of invasive carcinoma (IC) in hematoxylin-eosin (HE) slides.
- Locating them in associated HER2 slides.
- Evaluating the intensity and percentage of staining of HER2 within the IC regions.
- Using these scores to classify tumors as HER2-negative (0 or 1+), HER2-equivocal (2+), or HER2-positive (3+).
- Further confirming HER2 status in equivocal cases using additional testing methods like fluorescence in situ hybridization (FISH).
This process is tedious and time consuming. It has also proven to hold inter-observer variability. AI-based systems could efficiently assist pathologists in a more accurate clinical diagnosis.
Material & Method
Datasets & training
IC detection. Train EfficientNet on HE.
Registration process. Using VALIS package.
- WSIs normalization
- Features matching.
- Image ordering such that each WSI is adjacent to its most similar WSI.
- Rigid and non-rigid transformations.
Patch classification. Train classifier on x10 HER2 patches.
Inference pipeline
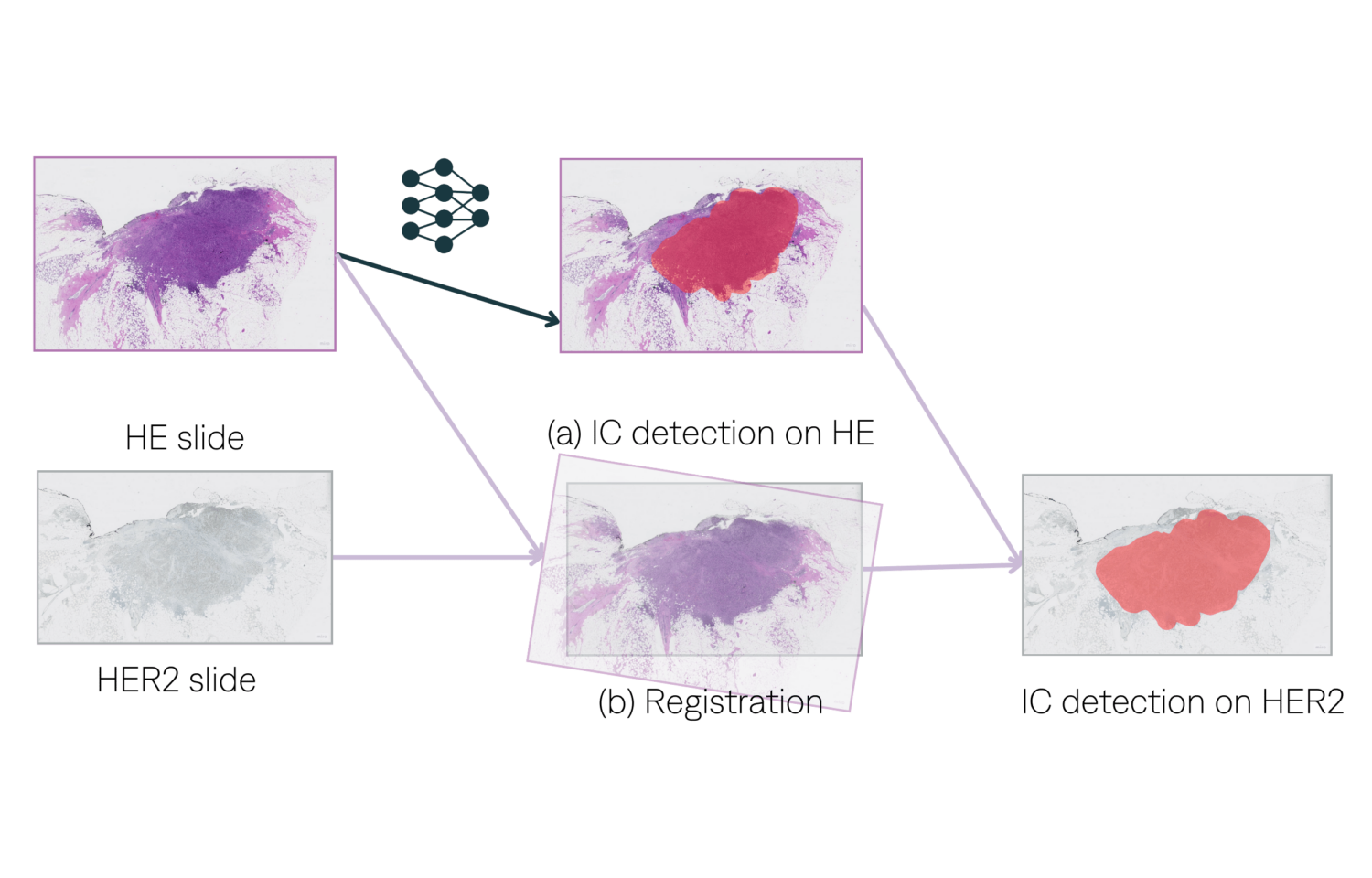
Experiments & results
IC detection
The detection results for IC show that using HE with registration achieved an F1-score of 83%, a recall of 88%, and a precision of 79% across 167 slides. In contrast, using HER2 alone resulted in an F1-score of 73%, a recall of 79%, and a precision of 68%.
HER2 class determination
To evaluate performance, four pathologists independently assessed 155 WSIs with varying HER2 expression levels. Ground truth scores were determined by the majority score assigned among pathologists. We observed moderate inter-observer agreement, with a mean Cohen’s Kappa coefficient of k=0.62. To measure scoring quality, we assessed the accuracy and provide the corresponding confusion matrix.
Discussion
For the IC detection task, HE-IHC registration is more efficient than detecting the tumor on IHC, but more annotated data IHC could correct this.
For the three-grade HER2 classification task, our pipeline achieved an accuracy of 89.7%. Remarkably, it accurately distinguished positive slides with only one misclassification, which occurred for a slide where pathologists had low concordance. Additionally, other misclassifications primarily involved negative slides categorized as 2+, minimally impacting diagnostic accuracy. Furthermore, our classification performs better on a subgroup of slides where pathologists have the highest agreement, achieving an accuracy of 93.3%.
Conclusion
Our study presents a deep learning pipeline for HER2 expression classification in breast samples. Evaluated with four pathologists, it showed a 89.7% accuracy in three-grade classification with main errors found within WSIs of uncertain score. Despite inter-observer variability, the pipeline aligns well with pathologists’ assessments, highlighting its potential for accurate HER2 slide scoring. These findings underscore its value as a reliable tool for pathological data analysis, with implications for enhancing breast cancer diagnosis and treatment planning.