SCIENTIFIC PUBLICATION
Multicenter Automatic Detection of Invasive Carcinoma on Breast Whole Slide Images
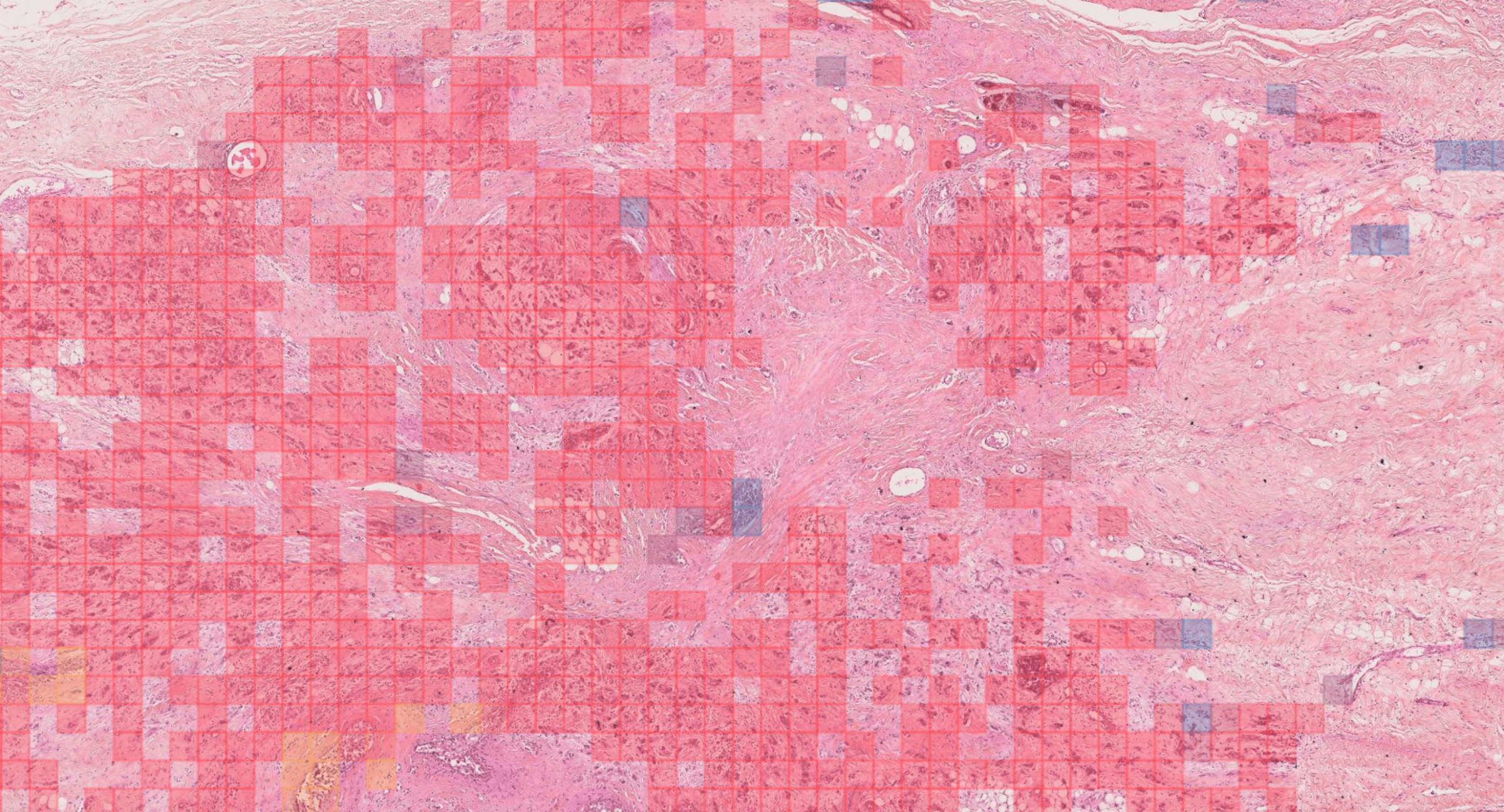
Rémy Peyret PhD, Nicolas Pozin PhD, Stéphane Sockeel PhD, Solène-Florence Kammerer-Jacquet MD, Julien Adam MD-PhD, Claire Bocciarelli MD, Yoan Ditchi MD, Christophe Bontoux MD, Thomas Depoilly MD, Loris Guichard MD, Elisabeth Lanteri MD, Marie Sockeel MD, Sophie Prévot MD-PhD.
ABSTRACT
Background
Breast cancer is one of the most prevalent cancers worldwide and pathologists are closely involved in establishing a diagnosis.
Cancer detection is a major public health issue, with almost 10 million cancer deaths worldwide in 2020, 19.3 million new cases diagnosed, and an expected rise to 28.4 million cases from 2020 to 2040 (+47%). Breast cancer (BC) has now surpassed lung cancer as the most commonly diagnosed malignancy (2.3 million new cases diagnosed worldwide, 11.7% of all cancer diagnoses) and is the leading or second cause of premature death in women in many countries according to the World Health Organization. Accurate and prompt detection of BC is essential to improve treatment efficacy and survival.
Tools to assist in making a diagnosis are required to manage the increasing workload. The average annual workload of pathologists has increased by around 5–10% and current data indicate a shortage of histopathologists worldwide leading to overwork, fatigue, and a higher risk of mistakes and diagnostic errors.
In this context, artificial intelligence (AI) and deep-learning based tools may be used in daily pathology practice. However, it is challenging to develop fast and reliable algorithms that can be trusted by practitioners, whatever the medical center.
Methods
We describe a patch-based algorithm that incorporates a convolutional neural network to detect and locate invasive carcinoma on breast whole-slide images. The network was trained on a dataset extracted from a reference acquisition center. We then performed a calibration step based on transfer learning to maintain the performance when translating on a new target acquisition center by using a limited amount of additional training data. Performance was evaluated using classical binary measures (accuracy, recall, precision) for both centers (referred to as “test reference dataset” and “test target dataset”) and at two levels: patch and slide level.
Findings
At patch level, accuracy, recall, and precision of the model on the reference and target test sets were 92.1% and 96.3%, 95% and 87.8%, and 73.9% and 70.6%, respectively. At slide level, accuracy, recall, and precision were 97.6% and 92.0%, 90.9% and 100%, and 100% and 70.8% for test sets 1 and 2, respectively.
Interpretation
The high performance of the algorithm at both centers shows that the calibration process is efficient. This is performed using limited training data from the new target acquisition center and requires that the model is trained beforehand on a large database from a reference center. This methodology allows the implementation of AI diagnostic tools to help in routine pathology practice.
Financial Disclosure
This study was carried out at Primaa, which is a startup company. The funders had no role in study design, data collection and analysis, decision to publish, or preparation of the manuscript. Two of the authors, M.S. and S.S., are co-founders and employees of this company, two authors, R.P. and N.P., are employees, and the rest are collaborators but did not receive any salary from Primaa.